๐คน๐ปโโ๏ธ ๊ฐ๋ ฅํ ์๊ฐํ library seaborn์ ์ธ ๊ฐ์ ๊ทธ๋ํ displot, pariplot, regplot์ ๋ํด์ ์์๋ณด๋ ์๊ฐ์ ๊ฐ์ ธ๋ณด๋ ค ํ๋ค.
๐ก seaborn.displot() docu
https://seaborn.pydata.org/generated/seaborn.displot.html
๐ก seaborn.pairplot() docu
https://seaborn.pydata.org/generated/seaborn.pairplot.html
๐ก seaborn.regplot() docu
https://seaborn.pydata.org/generated/seaborn.regplot.html
1> seaborn.displot
seaborn.displot(data=None, *, x=None, y=None, hue=None, row=None, col=None, weights=None, kind='hist', rug=False, rug_kws=None, log_scale=None, legend=True, palette=None, hue_order=None, hue_norm=None, color=None, col_wrap=None, row_order=None, col_order=None, height=5, aspect=1, facet_kws=None, **kwargs)
'This function provides access to several approaches for visualizing the univariate or bivariate distribution of data, including subsets of data defined by semantic mapping and faceting across multiple subplots.'
โ ํ ๊ฐ์ ๋ณ์ ๋๋ ๋ ๊ฐ์ ๋ณ์ ๊ฐ์ ๋ฐ์ดํฐ ๋ถํฌ๋ฅผ ์๊ฐํํ๋ displot() ํจ์์ด๋ค.
Data Analysis with Python (2/2) (from Coursera)
4) Model Development * A Model = a mathematical equation used to predict a value given one or more other values - Relating one or more independent variables to dependant variables (ex) 'high.....
sh-avid-learner.tistory.com
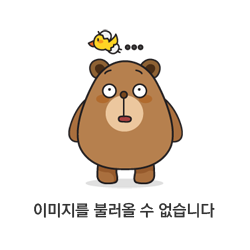
โ ์ coursera posting์์ ๋ ๋ณ์์ ๋ถํฌ๋ฅผ ํํํ๋ ๋ฐ seaborn์ distplot์ ์ฌ์ฉํ์๋๋ฐ, seaborn ๋ฒ์ ์ด ์ ๊ทธ๋ ์ด๋๋๋ฉด์ displot()์ผ๋ก ํํ๊ฐ ๋ฐ๋์๋ค. ์ ๊ทธ๋ ์ด๋๋๋ฉด์ ๋ ๋ค์ํ ํํ์ ๋ถํฌ๋ฅผ ์๊ฐํํ ์ ์๊ฒ ๋จ!
- univariate -
โ ์ธ์ kind์ ์ธ ๊ฐ์ ๊ฐ์ ๋ฃ์ ์ ์๋ค
โ 'hist(defalut)' - ํ์คํ ๊ทธ๋จ ์์ฑ
โก 'kde(kernel density estimates)' - ํ์คํ ๊ทธ๋จ์ ๊ฒ ํ ๋๋ฆฌ ์ ์ผ๋ก ๊ทธ์ ๊ทธ๋ํ๋ผ ์๊ฐํ๋ฉด ํธํ๋ค.
โข 'ecdf(empirical cumulative distribution functions)' - ๋์ ํ์คํ ๊ทธ๋จ์ ๋์ ๊ฐ์ ๋ํ๋ธ ๊ทธ๋ํ์ด๋ค. (univariate๋ง ํํ ๊ฐ๋ฅ)
โ ์ 1~3 ๊ทธ๋ํ ์์๋ฅผ ๋ณด๋ฉด ์๋์ ๊ฐ์ (์ผ์ชฝ๋ถํฐ histogram, kde, ecdf)
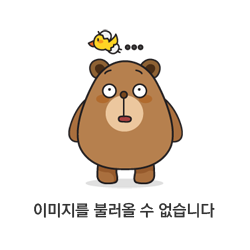
โ ํ์คํ ๊ทธ๋จ์ kde ๋ผ์ธ์ ์ถ๊ฐํด์ ๊ฒน์น ํํ๋ก ์๊ฐํ๋ ๊ฐ๋ฅ! (kde = True๋ก ์ธ์๋ฅผ ๋ฃ์ผ๋ฉด ๋จ)
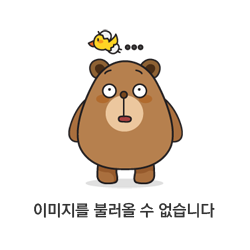
- bivariate -
โ default๋ก heatmap๊ณผ ๊ฐ์ ํํ๊ฐ ์ถ๋ ฅ๋๋ค (๋ ๋ณ์ ํน์ ๊ตฌ๊ฐ์ ํด๋น๋๋ count๊ฐ ๋์ผ๋ฉด ๋ ์งํ๊ฒ ์น ํด์ง๋ ํํ๋ก ์๊ฐํ๋จ!)
โก kind='kde'๊ฐ์ ๋ฃ์ผ๋ฉด ๋ฑ๊ณ ์ ์ ํํ๋ก ๋น๋๊ฐ ๋์ ์ชฝ์ ์ ์ด ๋ง์ด ๋ฐ์ง๋์ด ์๋ ํํ๋ก ์ถ๋ ฅ๋จ
(์ถ๊ฐ๋ก rug๊ฐ์ True๋ฅผ ๋ฃ์ผ๋ฉด ๊ธฐ์กด ๋ฑ๊ณ ์ ๊ทธ๋ํ์์ ๋ฐ์ง๋ data๋ฅผ ๊ฐ ์ถ ๋ณ๋ก ์ ์ ํตํด ๋ณด์ฌ์ค)
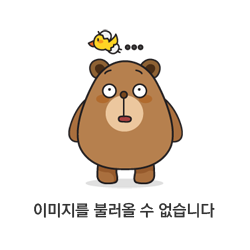
โ hue๊ฐ์ ํตํด ๋ค์ํ ๊ทธ๋ฃน์ data๋ฅผ ํ ๋ฒ์ ํ ๊ฐ์ ๊ทธ๋ํ๋ก ๋ถํฌ ๋น๊ต๋ฅผ ํ ์ ์๋ค๋ ์ ! (โป๋งค์ฐ ์ค์ ์ธ์) โ
โ multiple = 'stack' ๊ฐ์ ๋ฃ์ด ํ๋จ ์ฐ์ธก๊ณผ ๊ฐ์ด ๊ฒน์น ํ์คํ ๊ทธ๋จ ํํ๋ก๋ ํํ ๊ฐ๋ฅ (๋ฑํ ์ฌ์ฉํ์ง๋ ์์!)
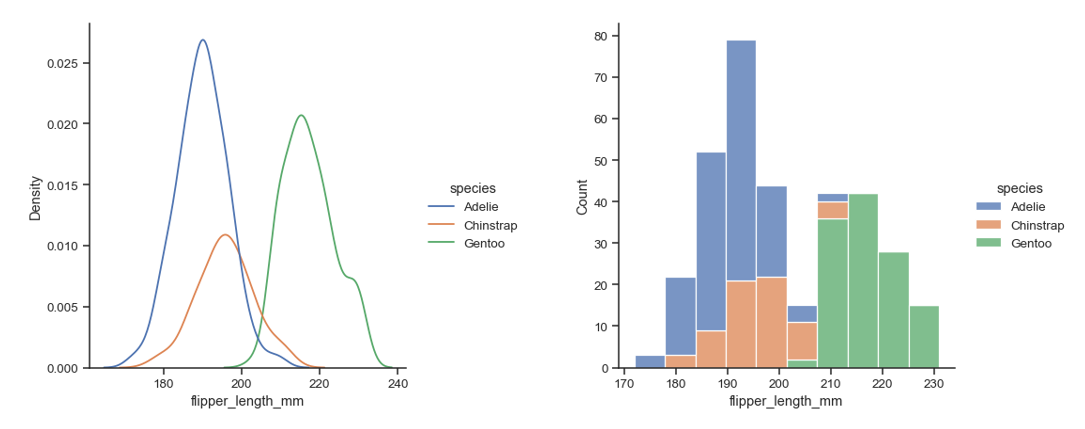
โ ํ ๋ฒ์ ์ฌ๋ฌ ๊ฐ์ ๊ทธ๋ํ, ์ฆ ๋ค์ํ facet ํํ๋ฅผ ์ถ๋ ฅํด์ฃผ์ด ์๊ฐํ๋ ๊ฐ๋ฅํ๋ค
โป ๋ค์ค ๊ทธ๋ํ๋ก ๋๋ ๊ธฐ์ค์ col ์ธ์๋ก ์ค์ ํด ๋๋ ์ ์์
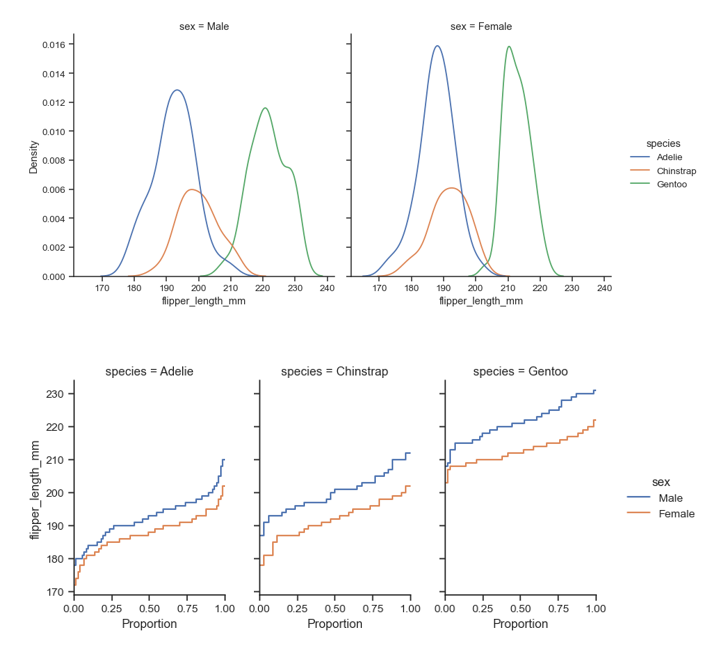
2> seaborn.pairplot
seaborn.pairplot(data, *, hue=None, hue_order=None, palette=None, vars=None, x_vars=None, y_vars=None, kind='scatter', diag_kind='auto', markers=None, height=2.5, aspect=1, corner=False, dropna=False, plot_kws=None, diag_kws=None, grid_kws=None, size=None)
'Plot pairwise relationships in a dataset. By default, this function will create a grid of Axes such that each numeric variable in data will by shared across the y-axes across a single row and the x-axes across a single column. The diagonal plots are treated differently: a univariate distribution plot is drawn to show the marginal distribution of the data in each column. It is also possible to show a subset of variables or plot different variables on the rows and columns.'
โ ์์์ ๋ฐฐ์ด displot์ ๊ฒฝ์ฐ ๋จ์ํ ๋ถํฌ ์์ฒด๋ฅผ ์ ์ ์๋ ์๊ฐํ์์ง๋ง, pairplot์ ๊ฒฝ์ฐ ๋ ๋ณ์๋ผ๋ฆฌ์ ๊ด๊ณ๋ ์ ์ ์๋ค. ์ถ๊ฐ๋ก, ์ผ์ชฝ ๋๊ฐ์ ์ ๋์ธ ๊ทธ๋ํ๋ค์ ๊ฐ ๋ณ์๋ณ ๋ถํฌ๋ฅผ ๋ํ๋ธ ๊ฒ์ผ๋ก, displot๋ณด๋ค ํจ์ฌ ๋ ๋ง์ ๊ธฐ๋ฅ์ ์ ๊ณตํ๋ ์๊ฐํ plot์ด๋ผ๊ณ ํ ์ ์์!
โ ๋ ๋ณ์ ๊ฐ์ ๊ด๊ณ๋ฅผ ๋ํ๋ด๋ ๊ทธ๋ํ๋ default๋ก scatterplot ์๊ฐํ๊ฐ ์ ๊ณต๋๋ฉฐ,
๋๊ฐ์ ํํ์ plot์ default histogram / hue๊ฐ์ผ๋ก ๊ทธ๋ฃนํํ๋ฉด kde ๊ทธ๋ํ๊ฐ ์ถ๋ ฅ๋๋ค
(์ด ๋, diag_kind๊ฐ์ ๋ค๋ฅธ ๊ทธ๋ํ ํ์ ์ hist๊ฐ์ผ๋ก ๋ฃ์ด์ ํ์คํ ๊ทธ๋จ์ผ๋ก๋ ์ถ๋ ฅ ๊ฐ๋ฅํ๋ค)
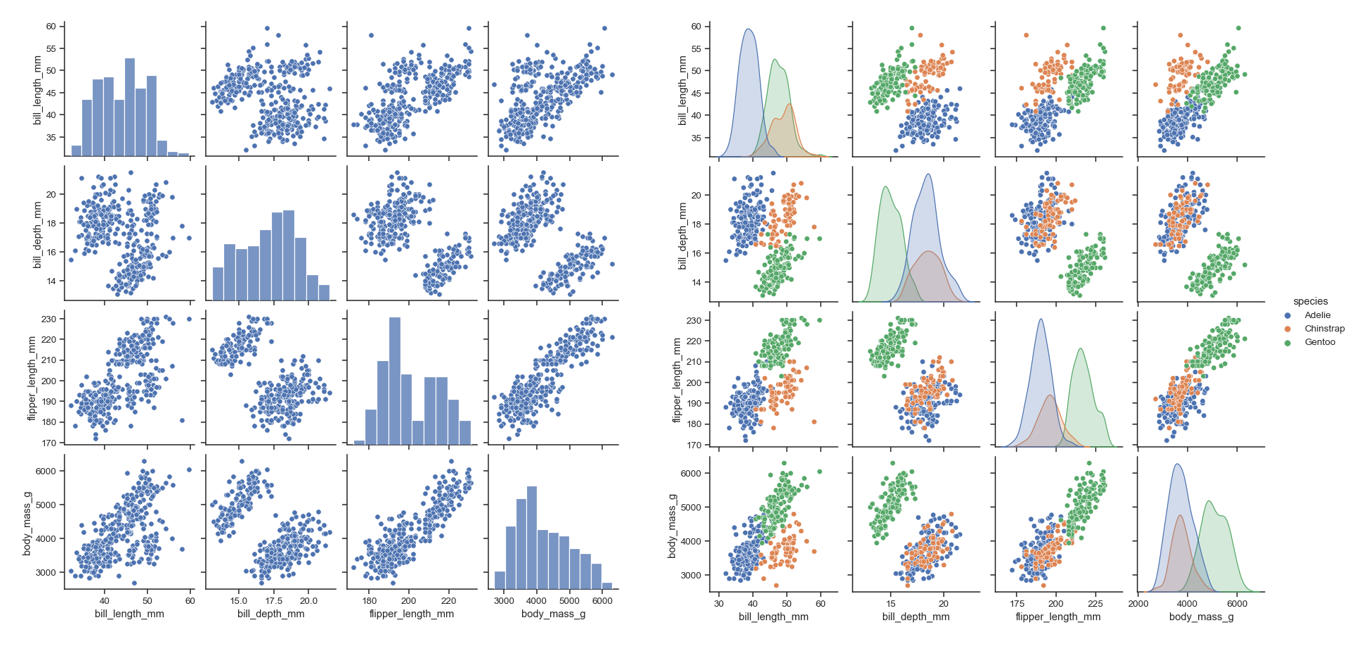
โ kind๊ฐ์ kde, hist๋ฅผ ๋ฃ์ด scatterplot์ด ์๋ ๋ค๋ฅธ ํํ๋ก ๋ ๋ณ์ ๊ฐ์ ๊ด๊ณ๋ฅผ ์๊ฐํํ ์๋ ์๋ค
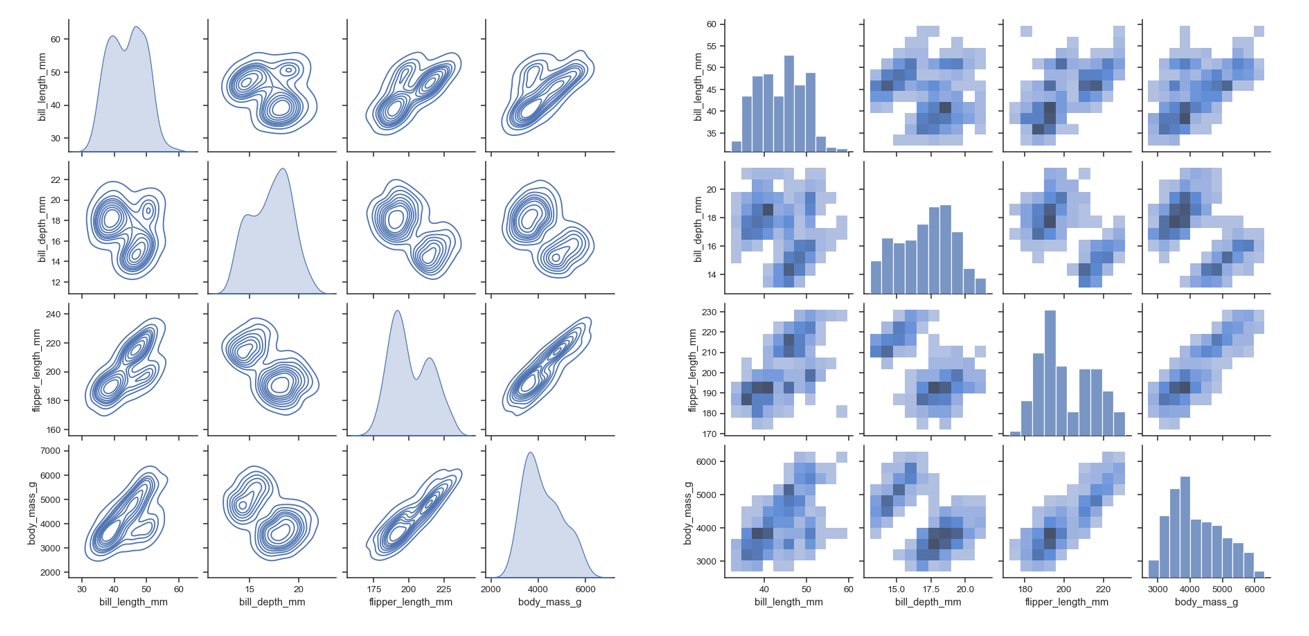
โ ๊ทธ ์ธ๋ก, x_vars์ y_vars์ ์ผ๋ถ ๋ณ์๋ง ์ง์ ํด ์ ์ฒด๊ฐ ์๋ pairplot์ ์ผ๋ถ๋ง ์๊ฐํ๋ ๊ฐ๋ฅํ๋ฉฐ,
markers๊ฐ์ ์ธ๋ถ์ ์ผ๋ก ์ค์ ํด scatterplot์ ์ ํํ๋ฅผ ๋ฌ๋ฆฌ ๋ฐ๊ฟ ์๋ ์์!
+ height๋ก ๊ฐ ์ธ๋ถ ๊ทธ๋ํ๋ค ๋์ด๋ ์ค์ ๊ฐ๋ฅ
+ corner = True๊ฐ์ผ๋ก ์ผ์ชฝ ๋๊ฐ์ ๊ธฐ์ค ์๋ ๊ทธ๋ํ (๋๊ฐ์ ํ๋กฏ ํฌํจ)๋ง ์๊ฐํ ์ค์ ๊ฐ๋ฅ
๊ทธ ์ธ๋ก dict ํํ๋ก ์ธ์๋ฅผ ์ง์ด๋ฃ์ด customize ๊ฐ๋ฅ!
์ถ๊ฐ์ ์ธ ๋ค์ํ ํํ๋ ์ดํ ํ๋ก์ ํธ์์ ์ฌ๋ฌ ์๊ฐํ๋ฅผ ์งํํ๋ฉด์ ์๊ฐํด ๋ณผ ์์ ์ด๋ค ๐โโ๏ธ
3> seaborn.regplot
seaborn.regplot(*, x=None, y=None, data=None, x_estimator=None, x_bins=None, x_ci='ci', scatter=True, fit_reg=True, ci=95, n_boot=1000, units=None, seed=None, order=1, logistic=False, lowess=False, robust=False, logx=False, x_partial=None, y_partial=None, truncate=True, dropna=True, x_jitter=None, y_jitter=None, label=None, color=None, marker='o', scatter_kws=None, line_kws=None, ax=None)
'Plot data and a linear regression model fit.'
โ ์์ ์ linear regression ๋ชจ๋ธ์ ๋ํด์ ๋ฐฐ์ด ์ ์ด ์๋ค.
Simple Linear Regression (concepts)
** ์ฐ๋ฆฌ๋ ์ ๋ฒ์๊ฐ์ Supervised Learning - Regression - Linear Regression๊น์ง concepts์ ๋ํด ๋ฐฐ์ ๋ค (โโโโโโ ํ๋จ ํฌ์คํ ์ฐธ์กฐ โโโโโโ) ML Supervised Learning โ Regression โ Linear Regr.....
sh-avid-learner.tistory.com
โ ์ฃผ์ด์ง data๊ฐ ์์ผ๋ฉด data์ ์ถ์ธ๋ฅผ ๋ฐ์ํด ํ ๊ฐ์ ์ ์ผ๋ก ์๋์ผ๋ก ์๊ฐํ ํด์ฃผ๋ plot (coursera ํฌ์คํ ์์ ์๋์ ๊ฐ์ด ๋ฐฐ์)
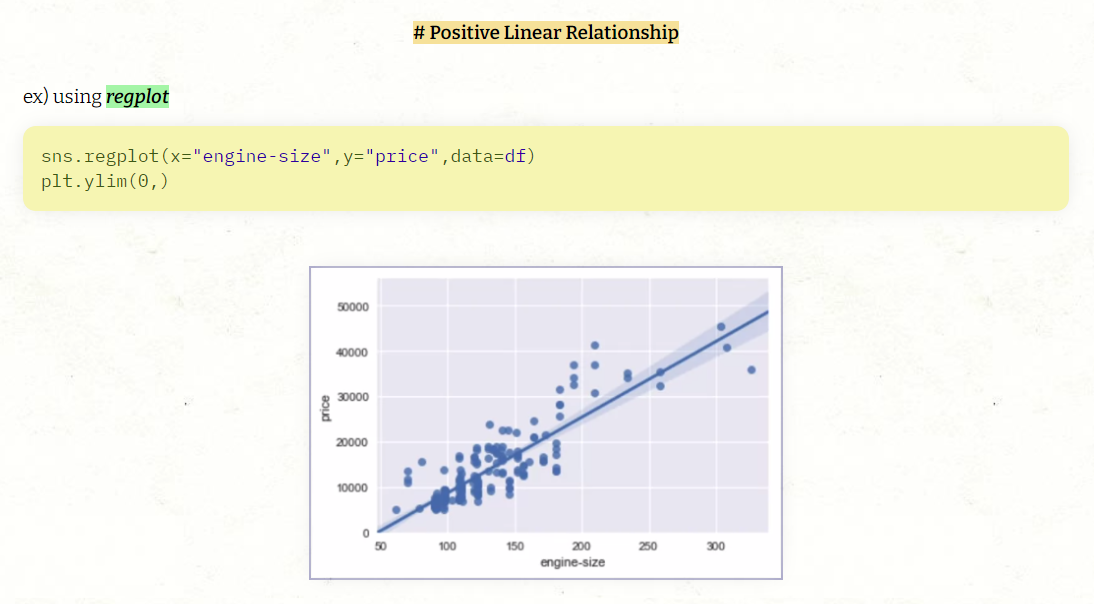
โ ์ผ๋ฐ์ ์ผ๋ก๋ ์๋ ์ข์ธก๊ณผ ๊ฐ์ด ๊ทธ๋ํ๊ฐ ํํ๋๊ณ , confidence interval ์์น๋ฅผ ๋ ์ค์ฌ์ ์ ์ด ํฌํจ๋๋ ๋ฒ์๋ฅผ ๋ ์ค์ฌ ๋ํ๋ผ ์ ์๋ค (์ฐ์ธก)
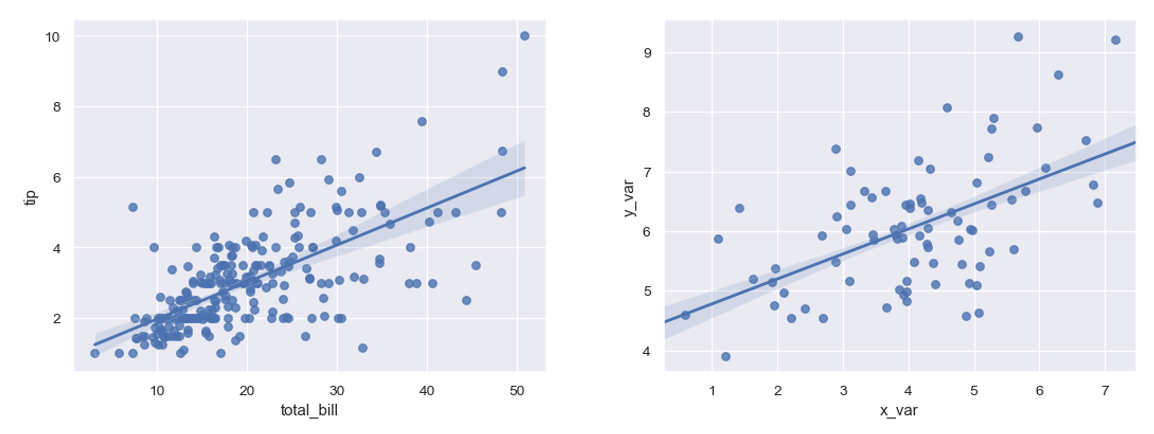
โ logistic ์ธ์๋ฅผ ์ค์ ํจ์ผ๋ก์จ ๋ก์ง์คํฑ ํ๊ท ๋ชจ๋ธ๋ก ์๊ฐํ๊ฐ ๊ฐ๋ฅํ๊ธฐ๋ ํจ!
๐คพ ์ด ์ธ ๊ฐ์ง์ seaborn plot์ ๋ํด ์์๋ณด์๋๋ฐ, ํ์ ์ง์ EDA ๊ณผ์ ๋๋ ๋ถ์๊ณผ์ ์์ ์ฌ๋ฌ ์๊ฐํ๋ฅผ ์ฌ์ฉํ ๋ ๋ง์ด ํ์ฉ์ด ๋ ๋ฏ ํ๋ค!
- ๊ฐ๋ ์ ๋ฆฌ ๋! ๐-
'Visualizations > Various Graphs' ์นดํ ๊ณ ๋ฆฌ์ ๋ค๋ฅธ ๊ธ
Visualization - Graphs summarized (0) | 2022.05.02 |
---|---|
violin plot (+seaborn) (0) | 2022.03.27 |
box plot (+seaborn) (0) | 2022.03.25 |
folium ์๊ฐํ (0) | 2022.03.24 |
๋๊ธ